
Six months ago I attended a webinar in which Ruha Benjamin asked, “What if we measured racism instead of race?” This challenge has weighed on me for several months as I have questioned when and why we collect information about the racial and ethnic identities of participants (and information about gender and disability) as we work towards educational equity.
Usually, we collect the demographic data to see if there are differentiated experiences or outcomes based on a participant’s racial or ethnic identity, gender, or disability status. In a large data set we can also look at intersectionality, recognizing that examining any one demographic variable alone often overlooks the ways in which marginalization can be compounded.
But, as Benjamin further challenged in that 2020 webinar, at what point are we expending resources to describe a problem we already know exists?
For example, I work on many projects seeking to broaden participation in computing. Demographic information is central to these efforts as we’re trying to reach students who are underrepresented in computing (those who aren’t white and/or Asian and male). Often, those who lack access to computing education also lack access to other educational opportunities, are in chronically under-resourced schools and/or are in schools that serve marginalized communities. So is it a surprise that in a school where students are not proficient in basic reading and math,[1] students also underperform in computing?
At what point are we expending resources studying a problem that we already know exists? And, by focusing on the racial and ethnic identities of students, are we ignoring the structural inequities in an inherently broken system?
These questions are challenging for me, as an external evaluator who is responsible for measuring progress against project goals but is not necessarily part of setting those goals. When the funder has goals explicitly tied to participant demographics, these challenging questions become impossible to avoid.
On one recent project, we’ve been addressing these questions by trying to capture structural inequities. We’re collecting information about the school districts in which equity-based educational interventions are being implemented. Specifically, we’re looking at urbanicity, median household income, and post-secondary education participation rates as compared to the national averages to better understand how the schools, and the communities in which they are situated, might be resourced. We also look at the profiles of the schools in which the interventions occur against the district as a whole. In this case recognizing the gaps in investment may help target additional project efforts.
As an evaluator, I always try to make my recommendations actionable. The data alone is not enough unless it is used to make meaningful changes to the program. I’m reorienting my work to include data on systematic factors beyond already-known racial demographics. Beyond providing information to the project team, this approach may help inform future resource allocation or policy changes. Without material impacts, evaluation is purely performative.
[1] Often measures of proficiency themselves have significant biases in which whiteness and class are rewarded. Using these types of measures also assumes a deficit model in which the students themselves, not the systems in which they’re educated, are the focus of the lack of deficiency.
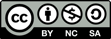
Except where noted, all content on this website is licensed under a Creative Commons Attribution-NonCommercial-ShareAlike 4.0 International License.